issue contents
March 2024 issue
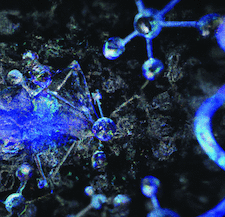
Cover illustration: In this issue, Billinge & Proffen [Acta Cryst. (2024), A80, 139–145] introduce a virtual collection of articles from various IUCr journals illustrating the application of artificial intelligence and machine learning in structural science. The cover image was generated by DALL·E using the prompt `A depiction of molecules surrounded by abstract representations of digital data and AI algorithms, highlighting the historical improvements in the data-driven approach to crystallography'.
editorial

advances
research papers

foundations
research papers





international union of crystallography

book reviews
