Navigation

- editorial
- research papers
- foundations
- research papers
- advances
- research papers
- foundations
- research papers
- advances
- research papers
- foundations
- research papers
- advances
- lead articles
- research papers
- CCP4
- feature articles
- research papers
- CCP4
- research papers
- CCP4
- research papers
- ccp4
- CCP4
- research papers
- short communications
- research papers
- short communications
- research papers
- computer programs
- research papers
- computer programs
- research papers
issue contents
Machine learning in crystallography and structural science
Edited by Simon J. L. Billinge and Thomas Proffen
This virtual collection gathers together articles from various IUCr journals, illustrating the application of artificial intelligence and machine learning in structural science. [A related virtual collection of recent articles in Journal of Applied Crystallography is also available at https://journals.iucr.org/special_issues/2024/ANNs/.]
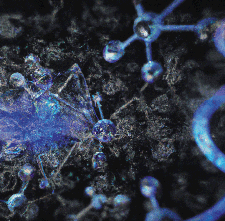
Cover illustration: An image generated by DALL·E using the prompt `A depiction of molecules surrounded by abstract representations of digital data and AI algorithms, highlighting the historical improvements in the data-driven approach to crystallography'.
Free 

An overview of the virtual collection on machine learning (ML) in crystallography and structural science, as represented in Acta Crystallographica Sections A, B and D, IUCrJ and Journal of Synchrotron Radiation, is presented. Some terms and concepts related to artificial intelligence and machine learning are briefly introduced and described, and a short history of ML in structural science as it appeared in these IUCr journals is given to whet the appetite for the rest of the collection.
It is shown how Voronoi polyhedra for C and H atoms in polynuclear aromatic hydrocarbons display characteristic differences that allow perfect atom-type prediction from knowledge of the atomic coordinates alone.
The indirect Fourier transform is discussed in the context of complementary statistical inference frameworks in order to determine a solution objectively, which then allows one to automate model-free analysis of small-angle scattering data. Modern machine-learning methods are used to obtain the most robust solution.
Download citation

Download citation

Open
access

In situ single-crystal X-ray diffraction data were used to unravel the structural dynamics and enthalpy and entropy of adsorption of CO2 into Y zeolite. A principal-component-analysis- (PCA) based approach is applied in an innovative way to single-crystal X-ray diffraction data analysis, allowing one to selectively detect the information from the subset of active atoms. The potential of and limitations of PCA in single-crystal diffraction are discussed.
CCDC reference: 1884781
We present applications of machine learning models for predicting the space group of the underlying structure from its atomic pair distribution function (PDF).
Open
access

The a posteriori probability densities of anomalous structure-factor amplitude differences were estimated by the Markov chain Monte Carlo machine-learning method. The model incorporated the correlation between the different Bijvoet pairs and the improved estimates were shown to be beneficial for SAD phasing.
PDB references: human survivin, 6sho; hen egg-white lysozyme, continuous rotation data collection and multivariate analysis of Friedel pairs, 6sij; hen egg-white lysozyme, continuous rotation, univariate, 6sik; hen egg-white lysozyme, inverse-beam geometry data collection and multivariate analysis of Friedel pairs, 6sil; hen egg-white lysozyme, inverse-beam geometry, univariate, 6sim
A novel data-driven approach for synchrotron Laue X-ray microdiffraction scans is presented based on machine learning techniques.
Open
access

Structure-mining finds and returns the best-fit structures from structural databases given a measured pair distribution function data set. Using databases and heuristics for automation, it has the potential to save experimenters a large amount of time as they explore candidate structures from the literature.
Open
access

A new web platform is presented for the pair distribution function (PDF) community to use and share advanced PDF analysis software in the cloud.
Open
access

A prototype application, pyDataRecognition, is described and tested. It has the goal that, given a measured powder diffraction pattern, it will return a list of publications from the IUCr Journals database that might be related based on the similarity to powder diffraction data deposited for those publications. This explores the possibility of a machine-readable literature where, for example, relevant studies may be found automatically through data similarity matches of online databases.
Machine learning was employed on the Cambridge Structural Database to derive a general force field for all observed atom–atom interactions. The force field parameters, i.e. interatomic potentials and `critical bond distances', are derived to calculate the intermolecular Gibbs energy, which is important for the prediction of crystal structures, solubility and other thermodynamic properties.
Open
access

Four deep learning architectures were applied and SqueezeNet scored best. It was combined with the grid programming system BOINC to realize automatic real-time scoring of crystallization well images. Scores are written to a database and displayed to facilitate image inspection for users.
Open
access

Neural networks were trained for robust classification of narrow electron beam diffraction patterns and may significantly decrease the need for storage space.
Open
access

Deep learning applications are increasingly dominating many areas of science. This paper reviews their relevance for and impact on protein crystallography.
This article explores gradient tree boosting based machine learning methods for classifying whether a particular ABO3 chemistry forms a perovskite or non-perovskite structured solid. A 95% test set classification accuracy is shown to be achieved for the best performing feature set.
A machine learning approach is developed to rapidly predict the displacements of previously unexplored octahedral cations in PbTiO3-based ferroelectric perovskites. Thus, expanding the knowledge base on ionic displacement data, which are important for the rational design of novel ferroelectric perovskites.
A new computational procedure called CAPRA is described that predicts coordinates of Cα atoms in density maps and outputs chains of Cα atoms representing the backbone of the protein.
Methodologies are presented for systematizing and representing knowledge about the chemical and physical properties of additives used in crystallization experiments. A novel machine-learning and discovery program is introduced as a method that uses such knowledge for automatic analysis of augmented macromolecular crystallization data in order to categorize and find interesting relationships that can potentially aid the growth of new crystals.
Open
access

A system for scoring images based on the likelihood of containing crystalline material is described. A simulation carried out on a real set of crystallization images demonstrates the utility of such a system in high-throughput environments by substantially reducing the manual workload necessary to detect crystals for X-ray screening.
Using an extended set of protein features calculated separately for protein surface and interior, a new version of XtalPred based on a random forest classifier achieves a significant improvement in predicting the success of structure determination from the primary amino-acid sequence.
Open
access

Recent advances in automated protein model building using ARP/wARP are presented. The new methods include machine-learning-enhanced sequence assignment and loop building using a fragment database.
Open
access

Two neural networks were trained to predict the correctness of protein residues by combining multiple validation metrics in Coot. Using the predicted correctness to automatically prune models led to significant improvements in the Buccaneer pipeline.
Open
access

The employment of directed acyclic graphs to advance the tracking, control and appraisal of crystallographic phasing strategies is discussed.
Open
access

This review discusses the AlphaFold2 system for protein structure prediction, including its conceptual and methodological advances, its amenability to interpretation and its achievements in the last Critical Assessment of protein Structure Prediction (CASP14) experiment.
The X-ray structure of BbgIII, a multidomain β-galactosidase from B. bifidum, determined using an intact protein corresponding to a gene construct of eight domains is reported.
PDB reference: BbgIII from Bifidobacterium bifidum, 7nit
Open
access

The implications of the AlphaFold2 protein structure-modelling software for crystallographic phasing strategies are discussed.
Open
access

A program utilizing artificial learning and convolutional neural networks, named Helcaraxe, has been developed which can detect ice-crystal artefacts in processed macromolecular diffraction data with unprecedented accuracy.
The new artificial intelligence-based protein structure modeling programs such as AlphaFold and RoseTTAFold have raised great enthusiasm in the scientific community. Here, it is shown that the excellent overall quality of these models can solve the phase problem faced by structural biology using X-ray diffraction. This study also validates these in silico models.
PDB reference: Kluyveromyces lactis Nmd4, 7qhy
Open
access

The use of AlphaFold2 predictions for the detection and correction of sequence-register errors among protein structures determined using cryo-EM deposited in the Protein Data Bank is described.
Open
access

A new protocol, DAQ-refine, for evaluating a protein model built from a cryo-EM map and applying local structure refinement is described.
Open
access

AlphaFold predictions can be used both as a starting point for structure determination and as a method of model optimization. The Phenix PredictAndBuild tool automates iterative prediction and model building, yielding a density map and model starting with sequence information and crystallographic data.
Open
access

A neural network trained to identify unfavourable fragments and therefore improve protein model building in the Buccaneer software is described.
Open
access

Emerging algorithms based on machine learning offer promise in processing new diffraction experiments.
Open
access

Artificial intelligence was used to characterize the diffraction in images from serial and rotation crystallography experiments. Forward simulations were used to train models to infer B factors, resolutions and the presence of crystal splitting from single diffraction images.
Protein crystal quality evaluation using cascade correlation neural networks.
The capabilities of artificial neural networks for the automatic and instantaneous analysis of nuclear resonant scattering spectra obtained at a synchrotron source are discussed.
Open
access

Use of convolutional neural networks for automated calibration of rotation axes is described.
Open
access

Deep learning provides one possible avenue to reduce the data stream generated by serial macromolecular X-ray crystallography. Convolutional neural networks can be trained to recognize the presence or absence of Bragg spots, forming a criterion to veto events prior to downstream data processing.
Open
access

A convolutional neural network has been designed to quickly and accurately upscale the sinograms of X-ray tomograms captured with a low number of projections, effectively increasing the number of projections. This is particularly useful for tomograms that are part of a time-series as, in order to capture fast-occurring temporal events, tomograms have to be collected quickly, requiring a low number of projections. The upscaling process is facilitated using a single tomogram with a high number of projections for training, which is usually captured at the end or the beginning of the time-series when capturing the tomogram quickly is no longer needed.
Open
access

An original and novel design scheme has been formulated to achieve an extremely high resolving power for a broad-band X-ray spectrometer with a relatively large source size, implementing a meridional pre-convex mirror to enhance the resolving power substantially while maintaining minimal intrinsic optical aberrations for the whole system to cast a decent flat-field at the detector domain throughout the spectral range.
Open
access

A fully automated crystal centering system using deep learning is presented. Using this system, a fully automated crystal structure determination pipeline has also been developed.
Open
access

A deep-learning method for limited angle tomography in synchrotron radiation transmission X-ray microscopies and a demonstration of its application in 3D visualization of a chlorella cell.
Open
access

A generative adversarial network (GAN) is used to reconstruct the missing-wedge tomographic data of an in situ ptychographic measurement.
Convolutional neural networks are useful for classifying grazing-incidence small-angle X-ray scattering patterns. They are also useful for classifying real experimental data.
Open
access

Unique transmission X-ray microscopy geometry allows high temporal resolution in absorption as well as phase contrast nanotomography. The evaluation of fast scan times versus image quality is presented.
This paper aims to develop a new method for training a deep neural network using synthetic data. The trained model will be used to automatically segment micro-CT images of bread dough collected at the Australian Synchrotron.
A parameter estimation method based on the deep learning CNN-LSTM model is proposed for overlapping nuclear pulses shaped by several exponential decay nuclear pulses.
A deep-learning-based image jitter correction method for synchrotron nano-resolution tomographic reconstruction with superior efficiency and accuracy is presented.
Open
access

A high-performance denoising filter based on machine learning for high-resolution synchrotron nanotomography data is analyzed and evaluated.
Open
access

A 3D U-net deep convolutional neural network has been developed and tested to segment precipitates in synchrotron-based X-ray tomography experiments. Comparison of predicted segmentation showed a good agreement with manual segmentation.
Open
access

AXEAP, a program that can process high-resolution emission spectrum data quickly, has been developed based on machine-learning algorithms.
Open
access

An approach based on machine learning to produce a fast-executing model is introduced that predicts the polarization and energy of the radiated light produced at an insertion device.
Open
access

The microscopy research at the Bionanoprobe (currently at beamline 9-ID and later 2-ID after APS-U) of Argonne National Laboratory focuses on applying synchrotron X-ray fluorescence techniques to obtain trace elemental mappings of cryogenic biological samples to gain insights about their role in critical biological activities.
Open
access

A framework for data-driven characterization of the nonlinear dynamics of a piezo-bimorph adaptive X-ray mirror has been developed. Rapid surface shape control and stability to within 2 nm RMS have been demonstrated.
Open
access

The capability of machine learning methods for identifying and separating artifacts that appear in a typical X-ray diffraction image is demonstrated.
Open
access

A new ring artifact correction method based on a residual neural network for tomographic reconstruction with superior efficiency and accuracy is presented.
Open
access

A new hybrid machine-learning approach for the automatic segmentation of dynamic computed tomography images during methane hydrate formation in sandy samples is presented. The algorithm allows for accurate and fast segmentation of gas hydrate changes and fluid flow in the low-contrast environment that is the main step to perform automatic quantitative analysis of processes in hydrate-bearing samples.
Open
access

A machine-learning-based closed-loop solution for reflectometry analysis in synchrotron beamline operation utilizing online data analysis is presented. This work focuses on the perspective of visiting facility users and strategies to provide an elementary data analysis in real time during the experiment without introducing the additional software dependencies in the beamline control software environment.
Open
access

This article proposes a deep-learning-based approach for synchrotron X-ray computed tomography with sparse-view projections. The experimental results indicate that tomographic images can be reconstructed by 75 X-ray projections without obvious streak artefacts and noise.
A deep-machine-learning technique based on a convolutional neural network (CNN) is introduced. It has been employed for the classification of crystal system, extinction group and space group for given powder X-ray diffraction patterns of inorganic materials.
Deep Consensus performs particle pruning in cryo-EM image-processing workflows using a smart consensus.
The performances of three image-classification algorithms were evaluated. The three classification methods lead to different datasets and subsequently result in different electron density maps of the reconstructed models.
A method (DeepRes) is presented to estimate a new local quality measure for 3D cryoEM maps that adopts the form of a `local resolution' type of information. DeepRes is fully automatic and parameter-free and avoids the issues of most current methods, such as their insensitivity to enhancements owing to B-factor sharpening, among others.
A self-supervised workflow uses a 2D class average to progressively train a convolutional neural network for automated particle picking in cryo-EM.
This paper describes a method for determining an atomic model of a protein complex using moderate-resolution cryoEM data and distance predictions from deep learning.
Twenty new Nd–Fe–B crystal structures can be found via the elemental substitution of 5967 host structures of lanthanides–transition metals–light elements collected from the Open Quantum Materials Database. The average atomic coordination number and coordination number of the Fe sites are the most important factors in determining the structure–stability relationship of the new substituted Nd–Fe–B crystal structures.
Machine-learning approaches can greatly facilitate single-particle-imaging experiments at X-ray free-electron-laser facilities by providing real-time images from the coherent X-ray diffraction data stream, using methods presented in this article.
The incorporation of prior knowledge about the structures of biological macromolecules into the reconstruction process of cryo-EM structure determination is proposed. Using a novel algorithm inspired by regularization by denoising, it is shown how convolutional neural networks can be used within this framework to improve reconstructions from simulated data.
A framework is described for the efficient and realistic simulation of X-ray diffraction scans to train machine- or deep-learning models like convolutional neural networks for the automatic phase-identification task in multiphase compounds.
A deep learning neural network has been developed to efficiently and accurately clean the background noise in experimental lab-based X-ray diffraction images. A better spot segmentation is obtained and thus grain mapping in 3D is improved compared with the conventional method.
findMySequence is presented – a machine-learning method for the identification of unknown proteins and sequence-assignment validation in cryo-EM and X-ray crystallography.
We propose BraggNN, a deep-learning based method, to accelerate the most computation-intensive part of polycrystal diffraction data analysis (diffraction signal characterization). The application of BraggNN for real experimental data demonstrates that it can deliver consistent (sometimes even slightly better) results compared with the conventional method while running hundreds of times faster.
A clustering algorithm based on deep learning is proposed to perform accurate image reconstruction from noisy coherent diffraction patterns. Structural changes in mitochondria induced by X-ray radiation damage are quantitatively characterized and analysed at the nanoscale with different radiation doses.
This article explores the use of the deep learning approach deepEMhancer as a regularizer in the RELION refinement process. deepEMhancer introduces prior information derived from macromolecular structures and contributes to noise reduction and signal enhancement, as well as a higher degree of isotropy, with a direct effect on image alignment and a reduction of overfitting during iterative refinement.
Smart Leginon leverages new machine-learning algorithms together with new microscope and image-handling algorithms to enable unattended, multi-grid cryoEM screening. Smart Leginon Autoscreen reduces microscope operator time from ∼6 h to <10 min for a typical session and recovers substantial idle microscope time outside of normal working hours.
Ptolemy uses deep learning and computer vision to automate multi-scale targeting in cryoEM data collection. It accurately finds and ranks targets of interest (`squares' and `holes') at successive magnification levels and generalizes across samples and microscopes.
Fast extraction of three-dimensional nanofiber orientation from WAXD patterns using machine learning
A machine-learning (ML) based framework can help to unveil the nanofiber orientation in hierarchically structured biological materials with the advantages of fast speed and automation. It promises to meet the analysis demand of extensive amounts of related data online and in real time in near-future next-generation synchrotrons.
Machine learning methods have been applied to solve simple Patterson maps. The results demonstrate the potential to use neural networks for solving the phase problem in crystallography.
A method to estimate crystal cell parameters directly from a pair distribution function profile, which makes use of machine-learning approaches combined with multivariate analysis and vector superposition techniques, is presented.